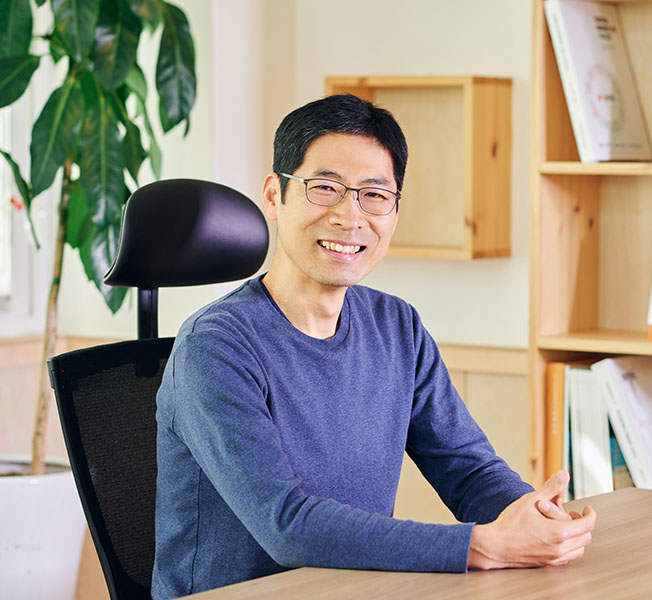
Changsin Lee l Tech Evangelist l Testworks
A special Meetup was organized by Testworks for developers and innovators who are interested in creating social values through technology. The first Meetup event occurred on June 17th, 2021. Unlike other meetups which went entirely online, the Meetup had a physical address and held in a Testworks conference room. Three people from three different companies presented their research results about using AI to help people with hearing impairment communicate.
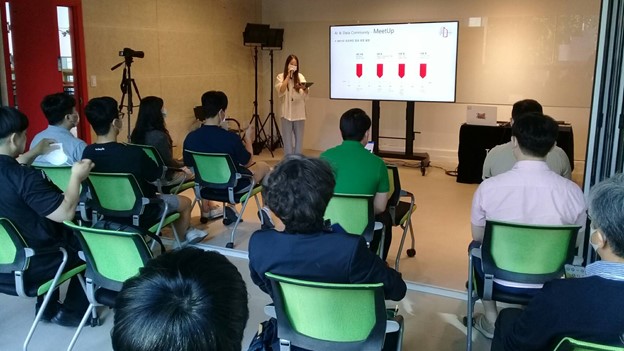
1. Sign language is naturally evolved: Contrary to my expectation, sign language is not invented by linguists, but naturally evolved just like any other natural language.
2. Not directly mappable to a natural language: Korean sign language is totally different from the Korean language. Similarly, American sign language cannot be replaced easily with English words. You must learn each sign language just like you are learning a foreign language.
3. More than hands: Hands are not the only media for expression. Equally important are NMS (non-manual signs) like facial expressions and body postures.
4. Lots of dialects and slangs: Surprisingly, there are many, many dialects, slang words, and personal idiosyncrasies in a sign language just like a natural language.
5. No standard written language: Unlike most natural languages, sign languages cannot be written down easily. In other words, there is no standard written language for sign language. This makes learning sign language difficult and training an AI system more challenging because you must capture the movements of signing in videos.
6. High illiteracy: You might think that people with hearing impairment can read lips or words so a sign language interpreter might not be necessary. Unfortunately, due to the disability, educational opportunities were limited so there are many who could not read or write their native natural language. Also, there is a lot of people with hearing impairment who do not understand sign language either.
There are 370,000 people with hearing impairment in Korea and only twelve percent understand written Korean language perfectly. With such a high illiteracy rate, sign language recognition through the help of AI has a huge social value. The goal is lofty but there are many technical challenges. The three talks showed some promising ongoing research results. Here is the summary:
Collecting Sign Language Key Points Using Multi-Cameras and 3D Data Augmentation
Seokmin Yun l Data Management Team Manager l Testworks
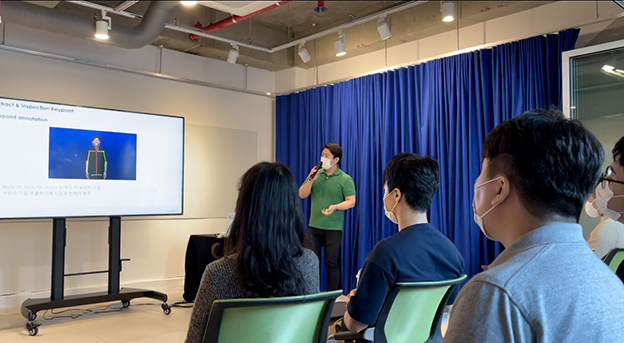
Collecting sign language data requires a special setup. Normal cameras cannot capture normal hand movements accurately due to slow shutter speed and occlusions can happen quite frequently. To collect good training data, five high speed cameras were used to capture the initial key points. Then 3D images were constructed which allowed to project key points from any angle or position. The setup required a careful calibration at the beginning and auto-annotation through openpose library was pivotal for the completion of the project.
Sign Language Recognition Using Multiple AI Systems
Han-Mu Park l Senior Researcher l KETI
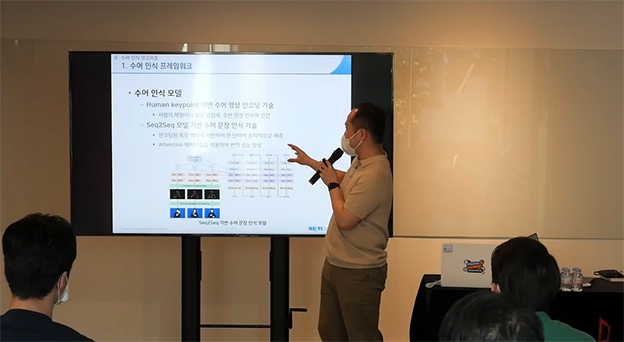
KETI developed its own Korean Sign Language recognition engine. The collection process differs from Testworks’ in that it used three ZED cameras but had to overcome similar technical challenges like pose estimation and occlusion. The pilot service project, however, showed promising results and used in Kimpo International Airport as a dedicated kiosk for sign language interpretation service. The current approach they took is to translate each sentence which is not scalable. The next version is to break it down to morpheme-level and enable dynamic composition of sentences.
Avatar Sign Language Generation Using AI
Mathew Huerta-Enochian l AI Developer l EQ4ALL
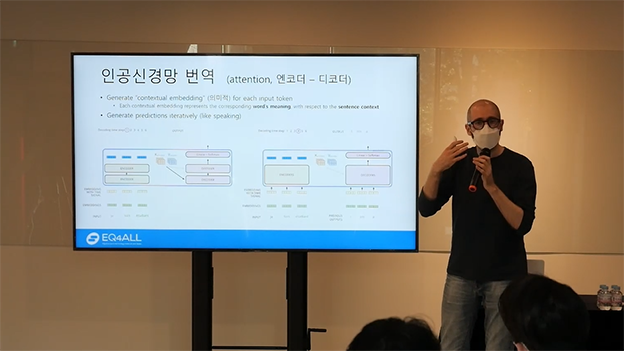
While Testworks and KETI focused on the collection and recognition aspect of sign language, EQ4ALL is working on the generation of sign language through Avatars. Leveraging the latest advancement in Deep Learning, especially Transformer-based NLP models, EQ4ALL turned generation of sign language from text into a neural machine translation problem. Using an attention-based encoder and decoder model, the model can generate real-time sign languages through an Avatar. The traditional symbolic methods (template-based and rule-based methods) are still used as a fallback mechanism, but the main work force is the attention-based neural machine translation.
Like any other AI projects, lack of sign language data is the biggest challenge that KETI and EQ4ALL face right now, and they are happy to see the quality data that Testworks was able to deliver.
More Meetups are scheduled for the future. If I take myself as an example, engineers have this nagging feeling in their minds wondering whether their work has any impact on other people. The forum might be a great vehicle for like-minded engineers. It was a small beginning, but it takes just a small spark to start a fire that burnt up the whole forest. I certainly saw a few scintillating lights tonight.