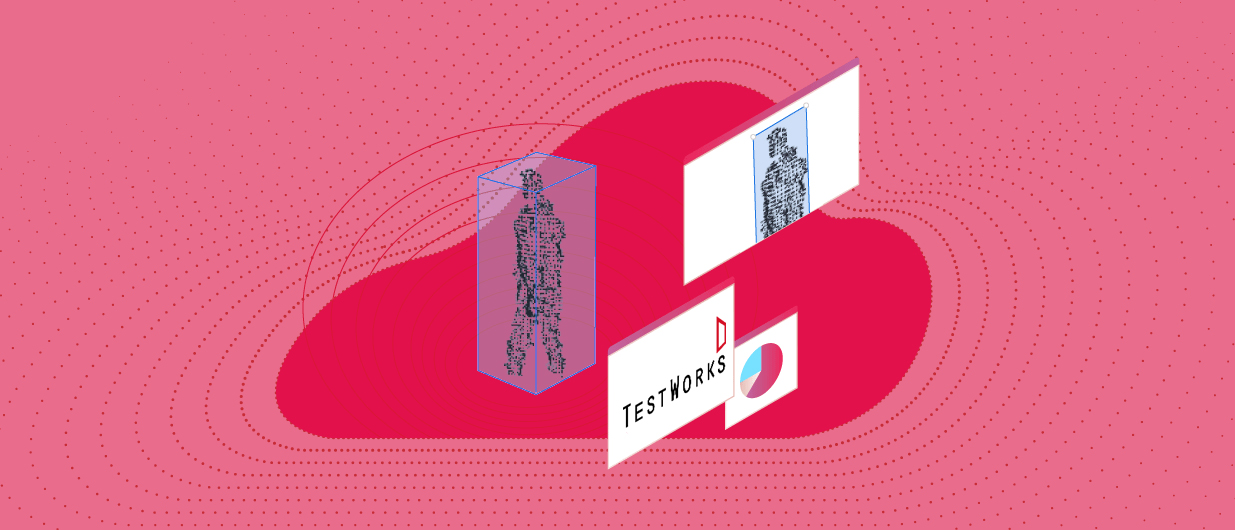
Overview
3D artificial intelligence, which deals with Point Cloud data in academia, has made remarkable progress in recent years. Thanks to this advancement, 3D artificial intelligence is currently being used in various industries such as autonomous driving, HD map production, robot driving, 3D scanning, and 3D contents development. 3D artificial intelligence is expected to make great contributions to each of these industry’s marketability, problem solving, and technological development in the near future.
However, the speed of each industry’s development in dealing with 3D artificial intelligence is still slower than expected. This is because the entry barrier to dealing with 3D data is considerably high compared to that of other forms of data. Expensive data collection equipment, 3D data’s format and standardization issue, and complex application software remain as a problem. The same hardship applies to building dataset for training artificial intelligence as well. In addition to the aforementioned problems, lackluster dataset processing tools and high difficulty of data post-processing and training for AI also remain. For this reason, the speed of building 3D datasets and developing artificial intelligence models that utilize such datasets are also at a slow pace.
Thus, there exists a need to resolve the high entry barrier of 3D Point Cloud data with adequate standardization guide that follows with it as well as providing comprehensible information to both companies and the public on 3D data processing and AI model training.
For this matter, our company Testworks is currently developing processing tools for Point Cloud data, which is one of primary 3D data formats. Through such effort, we seek to help people build 3D dataset much more conveniently than before.
Point Cloud Data Collection
Right now, due to the lack of refined standard to follow for Point Cloud data collection and processing along with its AI model, file storage formats and application software are highly inconsistent and incompatible. Therefore, some efforts are required to understand Point Cloud data and the information collected with them in addition to understanding its usage.
Information on point attributes
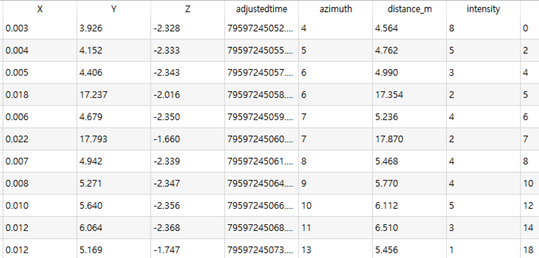
Each point of collected Point Cloud data contains spatial location information (x, y, z) and attribute information. Attribute information collected here varies from sensor to sensor, color information (R, G, B) collected through RGB-D cameras and reflectance intensity collected through LiDAR being primary examples. We need to collect them all, as most Deep Learning models that train from 3D data also utilize such attribute information as part of training.
Visualization
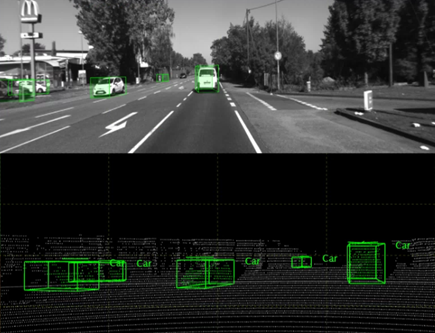
It is possible to visually represent the collected Point Cloud data in a three-dimensional space, but it is difficult to intuitively identify the object properties of points in the data with it alone. Labeling objects with corresponding type by workers are essential for artificial intelligence training, but it is difficult to do so just by looking at the dots. Therefore, it is essential to use a camera to collect images together to visually check object properties upon collecting Point Cloud data.
Sensor Synchronization
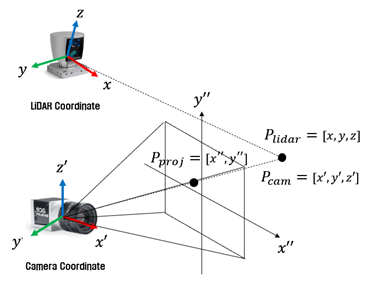
In order to train an artificial intelligence model based on Point Cloud data, at least two data collecting devices are required due to the visualization described above. Therefore, synchronization between the data collecting devices is essential. Synchronization involves time synchronization, which matches the collection time of sensors, and space synchronization, which matches the different coordinates between sensors. In the case of time synchronization, data must be collected while the sensors are time synchronized. In the case of spatial synchronization, coordinate transformation information for spatial synchronization work between collection equipment is stored.
Point Cloud Data Processing
The most significant concern of Testworks, a company specializing in building artificial intelligence datasets, is how to process Point Cloud data. Testworks is establishing a strategy on how to build and process training datasets at a time when the characteristics of Point Cloud data and the analysis of artificial intelligence models using Point Cloud data are completed. The processing work currently under consideration regarding Point Cloud dataset processing tools is as follows.
Point Cloud Segmentation
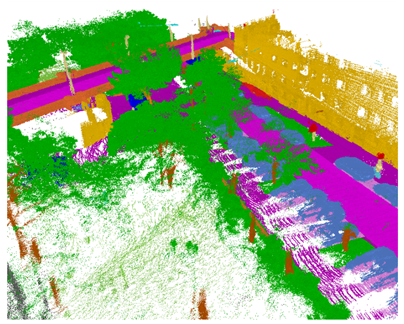
(Source: Excerpt from the paper Jens Behley, et al. “Semantic KITTI: A Dataset for Semantic Scene Understanding of LiDAR Sequences”)
The Point Cloud Segmentation model defines label information for all points within Point Cloud data, respectively. Since the number of data processing points for Segmentation learning is quite large, visualization of the data is essential, and label attributes must be assigned to each point in an effective way through processing tool.
3D Object Detection
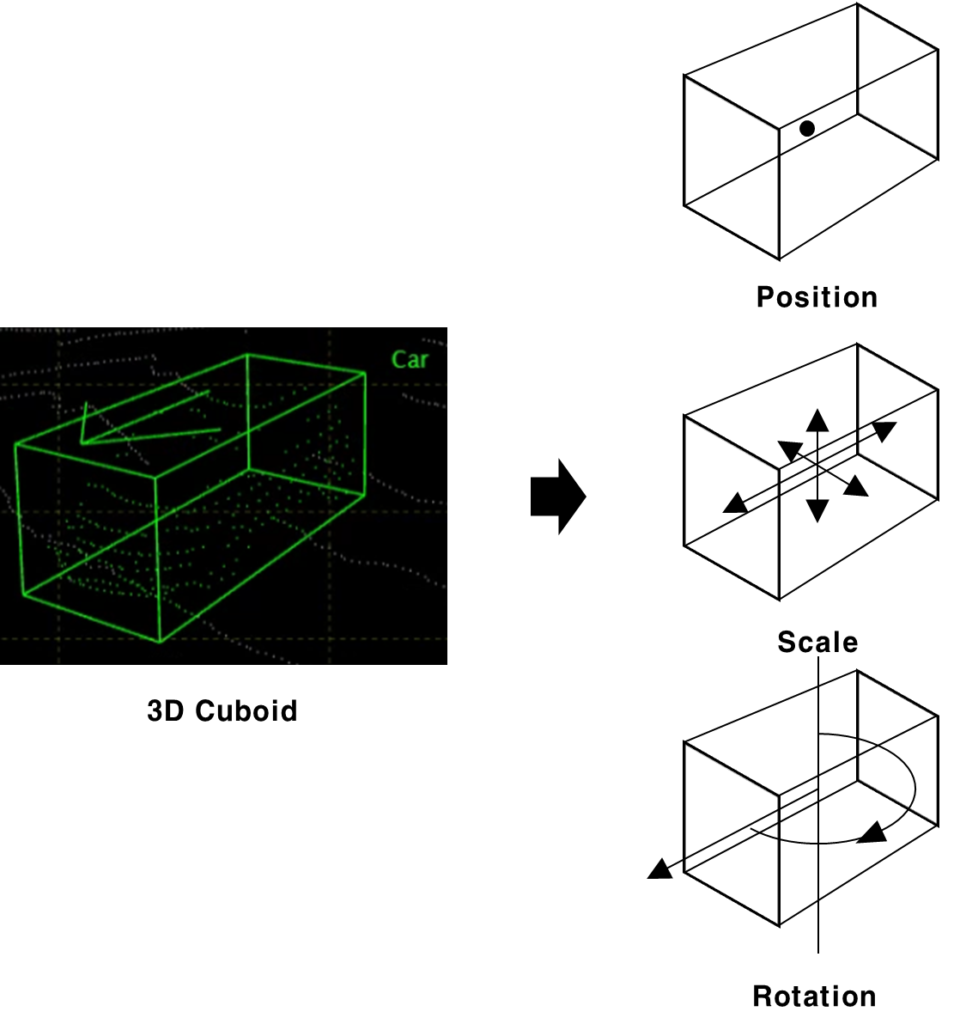
The 3D Object Detection model defines the properties of an object through a 3D Binding Box in the form of a rectangular parallelepiped. This 3D Bounding Box is also called a Cuboid. Cuboid information is generally defined in the ‘Position Scale Rotation’ (PSR) method, which is represented only by the position, scale, and rotation of the Cuboid. Therefore, it is effective to generate label data for dataset processing for training of the 3D Object Detection model.
Point Cloud Data Processing Automation
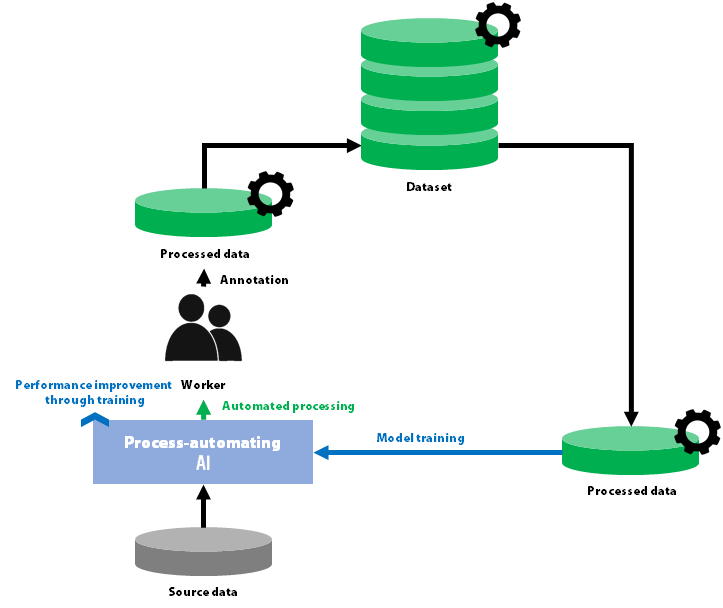
Although automation is already being performed in 2D image data processing, automation on 3D data processing also helps speed up the work. Testworks is conducting an artificial intelligence model training to help workers make the most of Point Cloud data-based artificial intelligence models.
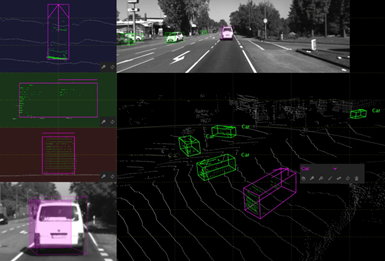
Summary
Artificial intelligence using 3D data has difficulties with development due to entry barriers such as expensive data collection equipment, application software including data set processing tools, and standardization issues. In 2019, Elon Musk, CEO of Tesla, criticized LIDAR for this matter. However, with the recent development of artificial intelligence models that overcome the limitations of Point Cloud data, attempts to develop artificial intelligence using 3D data are steadily increasing in various industries such as autonomous driving, HD map production, robot driving, 3D scanning, and 3D contents development. Testworks is a company specializing in artificial intelligence data construction and plans to continuously upgrade 3D data processing tools and automation models. Through this effort, we expect that artificial intelligence using 3D will be developed beyond 2D by accelerating the standardization of Point Cloud data and developing adequate application software.
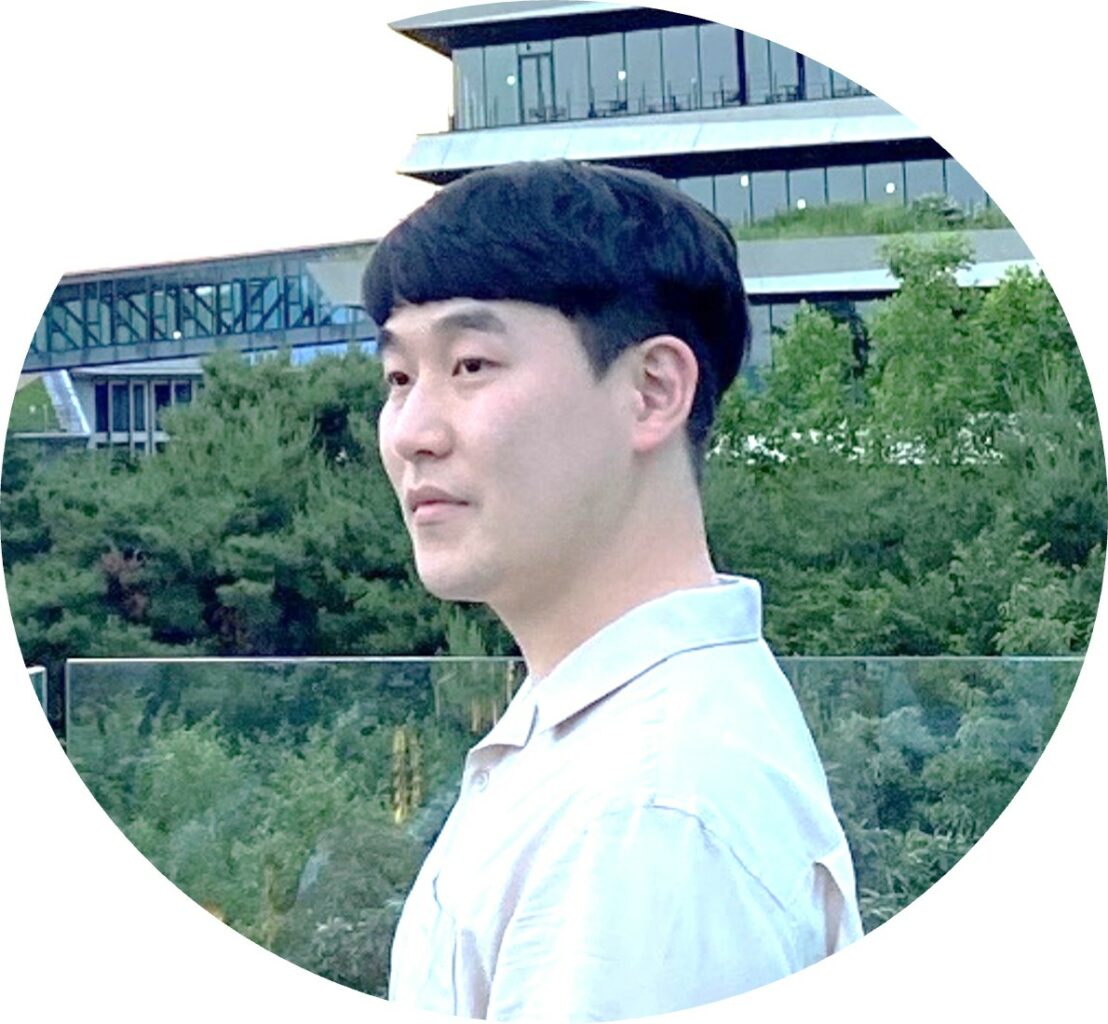
Yeseong Park
Researcher, AI R&D Team
BA, Mechanical System Design Engineering, Hongik University
MA, Intelligent Robotics, Graduate School of Hanyang University
He has been conducting research on robotics utilizing AI technology at the Intelligence Robot Research Institute of Hanyang University. He is working at AI R&D Team of Testworks with interest in computer vision and deep learning. He is now working on GAN, 3DAI studies.