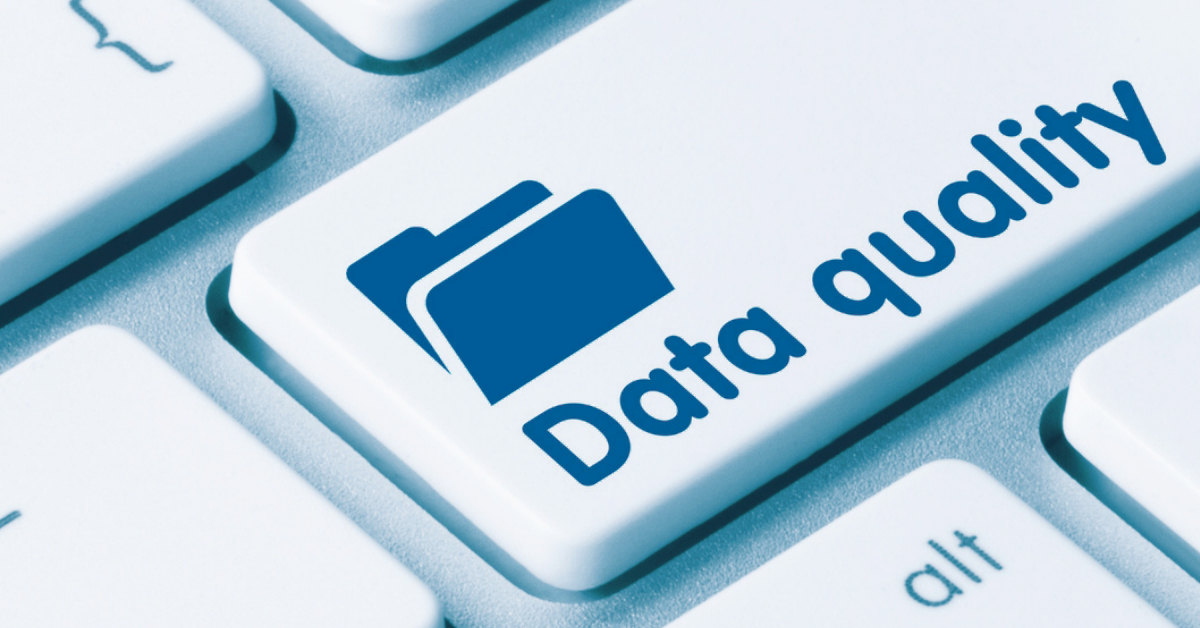
Wook Choi, Vice President and CPO | Changshin Lee, Director of AI TestOps Research | Hoyoung Byun, Model & Data QA Senior Researcher
The Importance of Data Quality
In order to train AI—the core of the 4th industrial revolution, AI researchers agree that a vast amount of data is necessary. However, a vast amount of data does not necessarily guarantee a high-performance AI, as can be seen with the recent example of Lee Luda, the AI trained with biased data, can cause ethical and social problems far beyond the performance problems. In order to develop a trustworthy AI service, the quality of the data used for its training is critical.
Up until today, AI development has been focused on model-centric approach and concentrated on how to better optimize the model with the same amount of data. Now, the development of ‘data-centric’ AI is mainly focusing on the quality of data that is being used. The importance of quality over quantity of data has been proven by countless experiments. In particular, considering the enormous cost of creating data, securing the minimum amount of quality data required for training will be far more ideal for the successful creation of AI data.
In order to bring about the age of AI services that we desire, a high-quality AI training data infrastructure must first be established. Through this blog series, we would like to introduce to you the importance of data quality and the necessary fields, quality tools and the element technology required.
The Difficulties of Data Quality Control
High quality service performance in the field of AI can only be achieved with high-quality data. To maintain consistent performance even after service deployment, continuous training of AI model is needed. This is due to continuous environmental changes and data biases discovered after deployment, which require adjustments to be made to improve the performance and other accuracy issues of AI-based services. Considering this, rigorous verification of training data quality is required at the time of its initial build and throughout the entire management. Starting with its initial build, the continuous collection of data and quality verification entails much effort and cost, which relies on the purpose of the AI training model. The difficulty in guaranteeing data quality that satisfies the purpose of an AI model lies in determining how data quality should be defined and measured. There have been many attempts to define data quality. A representative example is the Data Quality Control Guidelines for AI Training (v.1.0 2021. 2) published by the National Information Society Agency (NIA). The NIA presents the following quality indicators, as well as specific detailed indicators and the quality control guidelines for each indicator.
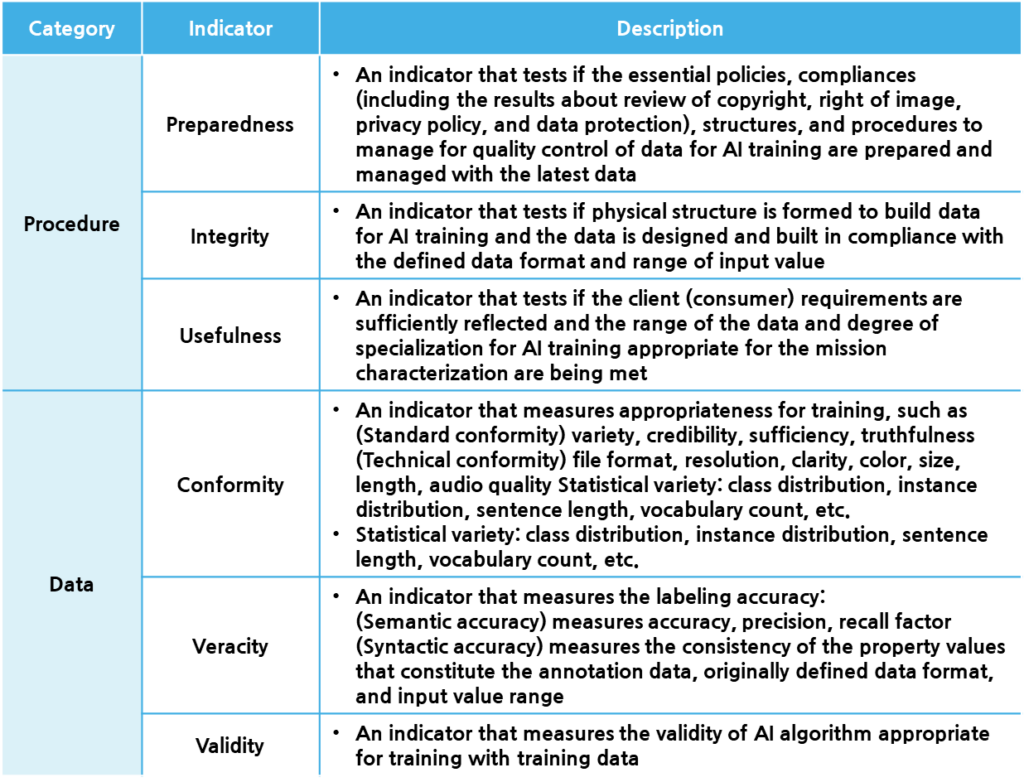
– Preparedness, integrity, and usefulness are quality indicators that are valid at the time of the build process in securing training datasets.
– Conformity and accuracy are quality indicators that are valid at the time of data labeling.
– Validity is a quality indicator that is valid at the time of the training model being provided.
When training an AI model, quality indicators that indicate the degree of suitability and the accuracy of data labeling are of utmost importance in ensuring reliable collection of various data so that such data can serve the purpose of the AI data. Accuracy quality indicators in the data labeling stage are divided into two sub-indicators: semantic accuracy and syntactic accuracy. Semantic accuracy is measured and verified by calculating accuracy, precision, and recall through comparison of annotations and ground truth (GT), while syntactic accuracy ensures that the annotation data structure is followed and that attribute values exist within the scope of input and conform to the defined data type.
Data Quality Verification Today
Most dataset building organizations use simple labeling tools internally developed or open-source/commercial tools to run projects. Of course, the quality of a dataset is improved by labeling the data, correcting errors, and conducting inspections. This is done by their workers inside the company, but it seems that no analysis of the actual errors is being made. Since the toolsused are specialized labeling tools designed for building dataset rather than quality verification, they are not as effective as verification tools in improving dataset quality. A competent data quality validation/verification tool should solve the following issues at the very least:
- Too many different formats exist because each company is building a dataset using their own customized format
- Separate conversion methods are used to validate each dataset format when performing data quality validation/verification on various training datasets
- The results of validation after quality verification for a large amount of data require manual creation of a verification report
- Reporting each object as an error in a task with many verification errors entails frustration
- It takes a large amount of time and effort when many objects are labeled in an image
- Sampling a reasonable amount of data from a target dataset stored in a folder for quality verification is a redundant task
- Difficulties lie in analyzing errors after verification due to the lack of a function to save error information separately
- If error analysis is not performed, the same mistakes will be repeated
- Repeated mistakes reduce efficiency and productivity
- There are difficulties with checking information related to statistical diversity of class or instance distribution and the uniformity/ratio of quantity for labeling verification results
There is apparently no commercial version of such a suitable tool available to solve the current difficulties in guaranteeing data quality. The labeling tools currently used by builders are different from this. To check the quality of datasets provided by multiple companies, the tools used by the respective companies must be downloaded and executed, and manual quality checks must be performed each time to report verification results. This requires much time and effort, but it is still not enough to guarantee the expected quality.
The First Fruit for Effective Quality Verification – The “Professional Tool for Data Quality Verification”
Seeing as domestic AI dataset construction projects are still in their infancy, the reality is that there are not many companies out there that can provide methodologies or tools that can quantitatively measure the data labeling quality indicators published by the National Information Society Agency (NIA). This is obvious as the data-driven development theory has only recently begun to attract attention, which is unfortunate for the many companies that were required to build and verify large amounts of data across various fields.
In light of this, Testworks started developing a quality verification tool that focuses on the accuracy and quality index of data labeling first and foremost while employing company strengths and expertise as a specialist in the field of AI data construction. Consequently, its beta version is due for release in mid-February 2022. As required functions for effective quality verification are to be determined, the first beta will focus on semantic accuracy, with a version that includes syntactic accuracy and verification automation to follow later.
As a company that prioritizes quality throughout the entire data construction process, Testworks will continue to think of ways to improve and advance data quality verification tools. We hope that our efforts will be of help to the builders of training datasets and quality verification companies both at home and abroad, and look forward to working together to develop quality tools, competitive at home and in overseas markets. Functional details of the data quality verification tool to be released by Testworks will be covered in the next series.